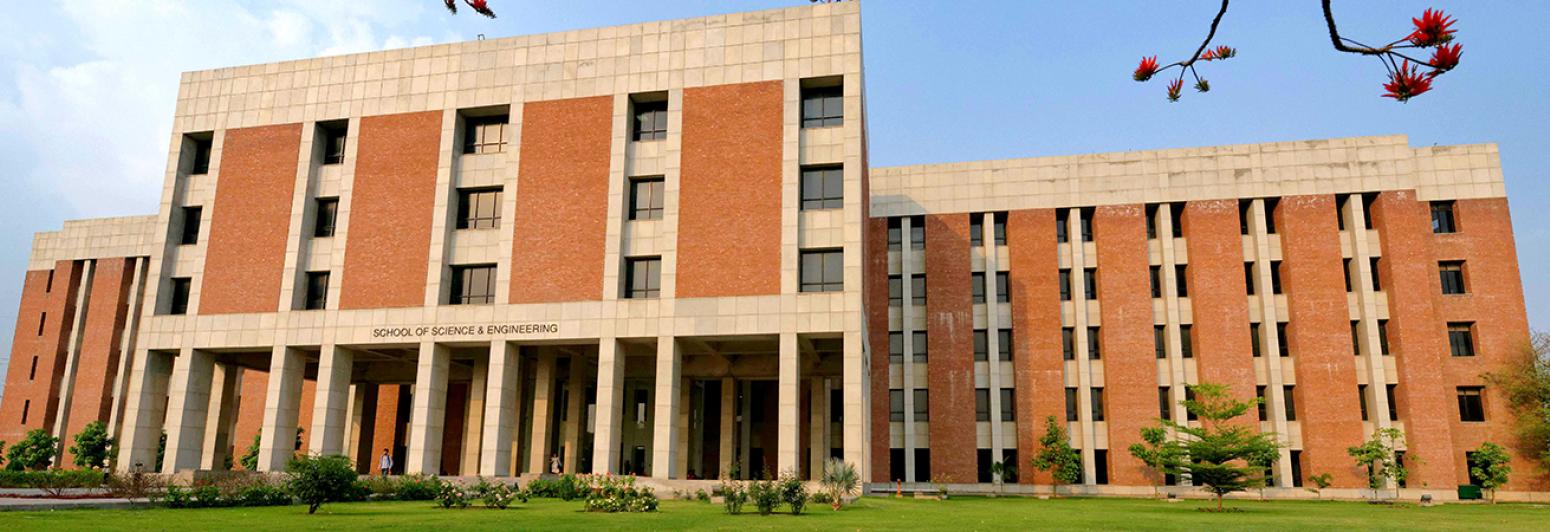
March 12, 2020 to June 12, 2020
Date: Friday, 12th June 2020
Time: 4:00 PM - 06:00 PM (Pakistan Standard Time)
Details for the Zoom Meeting
Link: https://zoom.us/j/99701418064?pwd=QmJpdWxEb3Q3OTR4d0hOMjBCY2VUZz09
Meeting ID: 997 0141 8064
Password: 667224
Final Defense Committee:
1. Dr. Naeem Ramzan (External Examiner), CS, University of the West of Scotland, UK.
2. Dr. Sohaib Khan (External Examiner), Hazen.ai, KSA
3. Dr. Asim Karim, CS, LUMS
4. Dr. Tariq Jadoon, EE, LUMS
5. Dr. Zubair Khalid, EE, LUMS
6. Dr. Murtaza Taj, CS, LUMS
Abstract:
Advancements in deep learning techniques beget a paradigm shift in computer vision especially some of its core problems for example content-based image retrieval (CBIR). It has a wide range of applications starting with scene recognition, digital image repository search, organization of image databases to 3D reconstruction. However, robust and accurate image retrieval from a large-scale image database in the field remote of sensing still remains an open problem. For particular multi-view image retrieval, challenges come not only from the severe visual overlap between the query image and irrelevant database images but also from geometric and photometric variations between the images taken from different views. Another obstacle affecting semantic image retrieval is large intra-class variation and inter-class similarity between semantic categories. In addition, recent supervised deep learning-based image representation enhances the performance of the existing framework at the expense of huge labelled image collections.
This research explores learning unsupervised visual descriptors in combination with deep metric learning (DML) as a replacement to conventional distance measurement for same-view and cross-view image retrieval (CVIR). For this purpose, multiple unsupervised visual representations and metric learning techniques are exploited through the introduction of novel deep models for both same-view and cross-view retrieval. Moreover, to avoid vanishing gradients and diminishing feature reuse problems inherited in deep models we propose a new residual unit termed as residual-dyad. Deep unsupervised features usually bear large memory footprints and are prone to the curse of dimensionality. Traditional feature pruning schemes involving aggregation of these learned visual descriptors lead to diminished performance. To resolve this in same-view retrieval, we also propose stacked autoencoder based solution to abbreviate unsupervised features without significantly affecting their discriminative and regenerative characteristics. Results demonstrate that our proposed solution achieves 25 times reduction in feature size with only 0.8 times the depletion of retrieval score.
Cross-view image retrieval being introduced for the first time is addressed through the development of a 9-class benchmark dataset named CrossViewRet. We leveraged the idea of cross-modal retrieval to handle cross-view retrieval through unsupervised as well as supervised visual representations. In addition, an adversarial feature learning technique (ADML) has also been proposed. This is adapted with an aim to find a feature space as well as a common semantic space in which samples from street-view images are compared directly to satellite-view images (and vice-versa). For this comparison, a novel deep metric learning-based solution has been proposed. Experimental evaluation illustrates the superiority of the proposed methods in the applications of same-view and cross-view image retrieval. We believe that introduction to a novel problem of CVIR task and the developed dataset would also serve as a baseline for future research.